mirror of
https://git.mirrors.martin98.com/https://github.com/langgenius/dify.git
synced 2025-04-23 22:29:49 +08:00
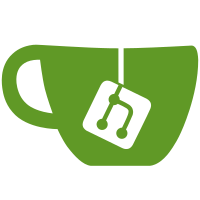
Signed-off-by: yihong0618 <zouzou0208@gmail.com> Signed-off-by: -LAN- <laipz8200@outlook.com> Signed-off-by: xhe <xw897002528@gmail.com> Signed-off-by: dependabot[bot] <support@github.com> Co-authored-by: takatost <takatost@gmail.com> Co-authored-by: kurokobo <kuro664@gmail.com> Co-authored-by: Novice Lee <novicelee@NoviPro.local> Co-authored-by: zxhlyh <jasonapring2015@outlook.com> Co-authored-by: AkaraChen <akarachen@outlook.com> Co-authored-by: Yi <yxiaoisme@gmail.com> Co-authored-by: Joel <iamjoel007@gmail.com> Co-authored-by: JzoNg <jzongcode@gmail.com> Co-authored-by: twwu <twwu@dify.ai> Co-authored-by: Hiroshi Fujita <fujita-h@users.noreply.github.com> Co-authored-by: AkaraChen <85140972+AkaraChen@users.noreply.github.com> Co-authored-by: NFish <douxc512@gmail.com> Co-authored-by: Wu Tianwei <30284043+WTW0313@users.noreply.github.com> Co-authored-by: 非法操作 <hjlarry@163.com> Co-authored-by: Novice <857526207@qq.com> Co-authored-by: Hiroki Nagai <82458324+nagaihiroki-git@users.noreply.github.com> Co-authored-by: Gen Sato <52241300+halogen22@users.noreply.github.com> Co-authored-by: eux <euxuuu@gmail.com> Co-authored-by: huangzhuo1949 <167434202+huangzhuo1949@users.noreply.github.com> Co-authored-by: huangzhuo <huangzhuo1@xiaomi.com> Co-authored-by: lotsik <lotsik@mail.ru> Co-authored-by: crazywoola <100913391+crazywoola@users.noreply.github.com> Co-authored-by: nite-knite <nkCoding@gmail.com> Co-authored-by: Jyong <76649700+JohnJyong@users.noreply.github.com> Co-authored-by: github-actions[bot] <41898282+github-actions[bot]@users.noreply.github.com> Co-authored-by: gakkiyomi <gakkiyomi@aliyun.com> Co-authored-by: CN-P5 <heibai2006@gmail.com> Co-authored-by: CN-P5 <heibai2006@qq.com> Co-authored-by: Chuehnone <1897025+chuehnone@users.noreply.github.com> Co-authored-by: yihong <zouzou0208@gmail.com> Co-authored-by: Kevin9703 <51311316+Kevin9703@users.noreply.github.com> Co-authored-by: -LAN- <laipz8200@outlook.com> Co-authored-by: Boris Feld <lothiraldan@gmail.com> Co-authored-by: mbo <himabo@gmail.com> Co-authored-by: mabo <mabo@aeyes.ai> Co-authored-by: Warren Chen <warren.chen830@gmail.com> Co-authored-by: JzoNgKVO <27049666+JzoNgKVO@users.noreply.github.com> Co-authored-by: jiandanfeng <chenjh3@wangsu.com> Co-authored-by: zhu-an <70234959+xhdd123321@users.noreply.github.com> Co-authored-by: zhaoqingyu.1075 <zhaoqingyu.1075@bytedance.com> Co-authored-by: 海狸大師 <86974027+yenslife@users.noreply.github.com> Co-authored-by: Xu Song <xusong.vip@gmail.com> Co-authored-by: rayshaw001 <396301947@163.com> Co-authored-by: Ding Jiatong <dingjiatong@gmail.com> Co-authored-by: Bowen Liang <liangbowen@gf.com.cn> Co-authored-by: JasonVV <jasonwangiii@outlook.com> Co-authored-by: le0zh <newlight@qq.com> Co-authored-by: zhuxinliang <zhuxinliang@didiglobal.com> Co-authored-by: k-zaku <zaku99@outlook.jp> Co-authored-by: luckylhb90 <luckylhb90@gmail.com> Co-authored-by: hobo.l <hobo.l@binance.com> Co-authored-by: jiangbo721 <365065261@qq.com> Co-authored-by: 刘江波 <jiangbo721@163.com> Co-authored-by: Shun Miyazawa <34241526+miya@users.noreply.github.com> Co-authored-by: EricPan <30651140+Egfly@users.noreply.github.com> Co-authored-by: crazywoola <427733928@qq.com> Co-authored-by: sino <sino2322@gmail.com> Co-authored-by: Jhvcc <37662342+Jhvcc@users.noreply.github.com> Co-authored-by: lowell <lowell.hu@zkteco.in> Co-authored-by: Boris Polonsky <BorisPolonsky@users.noreply.github.com> Co-authored-by: Ademílson Tonato <ademilsonft@outlook.com> Co-authored-by: Ademílson Tonato <ademilson.tonato@refurbed.com> Co-authored-by: IWAI, Masaharu <iwaim.sub@gmail.com> Co-authored-by: Yueh-Po Peng (Yabi) <94939112+y10ab1@users.noreply.github.com> Co-authored-by: Jason <ggbbddjm@gmail.com> Co-authored-by: Xin Zhang <sjhpzx@gmail.com> Co-authored-by: yjc980121 <3898524+yjc980121@users.noreply.github.com> Co-authored-by: heyszt <36215648+hieheihei@users.noreply.github.com> Co-authored-by: Abdullah AlOsaimi <osaimiacc@gmail.com> Co-authored-by: Abdullah AlOsaimi <189027247+osaimi@users.noreply.github.com> Co-authored-by: Yingchun Lai <laiyingchun@apache.org> Co-authored-by: Hash Brown <hi@xzd.me> Co-authored-by: zuodongxu <192560071+zuodongxu@users.noreply.github.com> Co-authored-by: Masashi Tomooka <tmokmss@users.noreply.github.com> Co-authored-by: aplio <ryo.091219@gmail.com> Co-authored-by: Obada Khalili <54270856+obadakhalili@users.noreply.github.com> Co-authored-by: Nam Vu <zuzoovn@gmail.com> Co-authored-by: Kei YAMAZAKI <1715090+kei-yamazaki@users.noreply.github.com> Co-authored-by: TechnoHouse <13776377+deephbz@users.noreply.github.com> Co-authored-by: Riddhimaan-Senapati <114703025+Riddhimaan-Senapati@users.noreply.github.com> Co-authored-by: MaFee921 <31881301+2284730142@users.noreply.github.com> Co-authored-by: te-chan <t-nakanome@sakura-is.co.jp> Co-authored-by: HQidea <HQidea@users.noreply.github.com> Co-authored-by: Joshbly <36315710+Joshbly@users.noreply.github.com> Co-authored-by: xhe <xw897002528@gmail.com> Co-authored-by: weiwenyan-dev <154779315+weiwenyan-dev@users.noreply.github.com> Co-authored-by: ex_wenyan.wei <ex_wenyan.wei@tcl.com> Co-authored-by: engchina <12236799+engchina@users.noreply.github.com> Co-authored-by: engchina <atjapan2015@gmail.com> Co-authored-by: dependabot[bot] <49699333+dependabot[bot]@users.noreply.github.com> Co-authored-by: 呆萌闷油瓶 <253605712@qq.com> Co-authored-by: Kemal <kemalmeler@outlook.com> Co-authored-by: Lazy_Frog <4590648+lazyFrogLOL@users.noreply.github.com> Co-authored-by: Yi Xiao <54782454+YIXIAO0@users.noreply.github.com> Co-authored-by: Steven sun <98230804+Tuyohai@users.noreply.github.com> Co-authored-by: steven <sunzwj@digitalchina.com> Co-authored-by: Kalo Chin <91766386+fdb02983rhy@users.noreply.github.com> Co-authored-by: Katy Tao <34019945+KatyTao@users.noreply.github.com> Co-authored-by: depy <42985524+h4ckdepy@users.noreply.github.com> Co-authored-by: 胡春东 <gycm520@gmail.com> Co-authored-by: Junjie.M <118170653@qq.com> Co-authored-by: MuYu <mr.muzea@gmail.com> Co-authored-by: Naoki Takashima <39912547+takatea@users.noreply.github.com> Co-authored-by: Summer-Gu <37869445+gubinjie@users.noreply.github.com> Co-authored-by: Fei He <droxer.he@gmail.com> Co-authored-by: ybalbert001 <120714773+ybalbert001@users.noreply.github.com> Co-authored-by: Yuanbo Li <ybalbert@amazon.com> Co-authored-by: douxc <7553076+douxc@users.noreply.github.com> Co-authored-by: liuzhenghua <1090179900@qq.com> Co-authored-by: Wu Jiayang <62842862+Wu-Jiayang@users.noreply.github.com> Co-authored-by: Your Name <you@example.com> Co-authored-by: kimjion <45935338+kimjion@users.noreply.github.com> Co-authored-by: AugNSo <song.tiankai@icloud.com> Co-authored-by: llinvokerl <38915183+llinvokerl@users.noreply.github.com> Co-authored-by: liusurong.lsr <liusurong.lsr@alibaba-inc.com> Co-authored-by: Vasu Negi <vasu-negi@users.noreply.github.com> Co-authored-by: Hundredwz <1808096180@qq.com> Co-authored-by: Xiyuan Chen <52963600+GareArc@users.noreply.github.com>
753 lines
33 KiB
Python
753 lines
33 KiB
Python
import concurrent.futures
|
|
import datetime
|
|
import json
|
|
import logging
|
|
import re
|
|
import threading
|
|
import time
|
|
import uuid
|
|
from typing import Any, Optional, cast
|
|
|
|
from flask import current_app
|
|
from flask_login import current_user # type: ignore
|
|
from sqlalchemy.orm.exc import ObjectDeletedError
|
|
|
|
from configs import dify_config
|
|
from core.entities.knowledge_entities import IndexingEstimate, PreviewDetail, QAPreviewDetail
|
|
from core.errors.error import ProviderTokenNotInitError
|
|
from core.model_manager import ModelInstance, ModelManager
|
|
from core.model_runtime.entities.model_entities import ModelType
|
|
from core.rag.cleaner.clean_processor import CleanProcessor
|
|
from core.rag.datasource.keyword.keyword_factory import Keyword
|
|
from core.rag.docstore.dataset_docstore import DatasetDocumentStore
|
|
from core.rag.extractor.entity.extract_setting import ExtractSetting
|
|
from core.rag.index_processor.constant.index_type import IndexType
|
|
from core.rag.index_processor.index_processor_base import BaseIndexProcessor
|
|
from core.rag.index_processor.index_processor_factory import IndexProcessorFactory
|
|
from core.rag.models.document import ChildDocument, Document
|
|
from core.rag.splitter.fixed_text_splitter import (
|
|
EnhanceRecursiveCharacterTextSplitter,
|
|
FixedRecursiveCharacterTextSplitter,
|
|
)
|
|
from core.rag.splitter.text_splitter import TextSplitter
|
|
from core.tools.utils.rag_web_reader import get_image_upload_file_ids
|
|
from extensions.ext_database import db
|
|
from extensions.ext_redis import redis_client
|
|
from extensions.ext_storage import storage
|
|
from libs import helper
|
|
from models.dataset import ChildChunk, Dataset, DatasetProcessRule, DocumentSegment
|
|
from models.dataset import Document as DatasetDocument
|
|
from models.model import UploadFile
|
|
from services.feature_service import FeatureService
|
|
|
|
|
|
class IndexingRunner:
|
|
def __init__(self):
|
|
self.storage = storage
|
|
self.model_manager = ModelManager()
|
|
|
|
def run(self, dataset_documents: list[DatasetDocument]):
|
|
"""Run the indexing process."""
|
|
for dataset_document in dataset_documents:
|
|
try:
|
|
# get dataset
|
|
dataset = Dataset.query.filter_by(id=dataset_document.dataset_id).first()
|
|
|
|
if not dataset:
|
|
raise ValueError("no dataset found")
|
|
|
|
# get the process rule
|
|
processing_rule = (
|
|
db.session.query(DatasetProcessRule)
|
|
.filter(DatasetProcessRule.id == dataset_document.dataset_process_rule_id)
|
|
.first()
|
|
)
|
|
if not processing_rule:
|
|
raise ValueError("no process rule found")
|
|
index_type = dataset_document.doc_form
|
|
index_processor = IndexProcessorFactory(index_type).init_index_processor()
|
|
# extract
|
|
text_docs = self._extract(index_processor, dataset_document, processing_rule.to_dict())
|
|
|
|
# transform
|
|
documents = self._transform(
|
|
index_processor, dataset, text_docs, dataset_document.doc_language, processing_rule.to_dict()
|
|
)
|
|
# save segment
|
|
self._load_segments(dataset, dataset_document, documents)
|
|
|
|
# load
|
|
self._load(
|
|
index_processor=index_processor,
|
|
dataset=dataset,
|
|
dataset_document=dataset_document,
|
|
documents=documents,
|
|
)
|
|
except DocumentIsPausedError:
|
|
raise DocumentIsPausedError("Document paused, document id: {}".format(dataset_document.id))
|
|
except ProviderTokenNotInitError as e:
|
|
dataset_document.indexing_status = "error"
|
|
dataset_document.error = str(e.description)
|
|
dataset_document.stopped_at = datetime.datetime.now(datetime.UTC).replace(tzinfo=None)
|
|
db.session.commit()
|
|
except ObjectDeletedError:
|
|
logging.warning("Document deleted, document id: {}".format(dataset_document.id))
|
|
except Exception as e:
|
|
logging.exception("consume document failed")
|
|
dataset_document.indexing_status = "error"
|
|
dataset_document.error = str(e)
|
|
dataset_document.stopped_at = datetime.datetime.now(datetime.UTC).replace(tzinfo=None)
|
|
db.session.commit()
|
|
|
|
def run_in_splitting_status(self, dataset_document: DatasetDocument):
|
|
"""Run the indexing process when the index_status is splitting."""
|
|
try:
|
|
# get dataset
|
|
dataset = Dataset.query.filter_by(id=dataset_document.dataset_id).first()
|
|
|
|
if not dataset:
|
|
raise ValueError("no dataset found")
|
|
|
|
# get exist document_segment list and delete
|
|
document_segments = DocumentSegment.query.filter_by(
|
|
dataset_id=dataset.id, document_id=dataset_document.id
|
|
).all()
|
|
|
|
for document_segment in document_segments:
|
|
db.session.delete(document_segment)
|
|
if dataset_document.doc_form == IndexType.PARENT_CHILD_INDEX:
|
|
# delete child chunks
|
|
db.session.query(ChildChunk).filter(ChildChunk.segment_id == document_segment.id).delete()
|
|
db.session.commit()
|
|
# get the process rule
|
|
processing_rule = (
|
|
db.session.query(DatasetProcessRule)
|
|
.filter(DatasetProcessRule.id == dataset_document.dataset_process_rule_id)
|
|
.first()
|
|
)
|
|
if not processing_rule:
|
|
raise ValueError("no process rule found")
|
|
|
|
index_type = dataset_document.doc_form
|
|
index_processor = IndexProcessorFactory(index_type).init_index_processor()
|
|
# extract
|
|
text_docs = self._extract(index_processor, dataset_document, processing_rule.to_dict())
|
|
|
|
# transform
|
|
documents = self._transform(
|
|
index_processor, dataset, text_docs, dataset_document.doc_language, processing_rule.to_dict()
|
|
)
|
|
# save segment
|
|
self._load_segments(dataset, dataset_document, documents)
|
|
|
|
# load
|
|
self._load(
|
|
index_processor=index_processor, dataset=dataset, dataset_document=dataset_document, documents=documents
|
|
)
|
|
except DocumentIsPausedError:
|
|
raise DocumentIsPausedError("Document paused, document id: {}".format(dataset_document.id))
|
|
except ProviderTokenNotInitError as e:
|
|
dataset_document.indexing_status = "error"
|
|
dataset_document.error = str(e.description)
|
|
dataset_document.stopped_at = datetime.datetime.now(datetime.UTC).replace(tzinfo=None)
|
|
db.session.commit()
|
|
except Exception as e:
|
|
logging.exception("consume document failed")
|
|
dataset_document.indexing_status = "error"
|
|
dataset_document.error = str(e)
|
|
dataset_document.stopped_at = datetime.datetime.now(datetime.UTC).replace(tzinfo=None)
|
|
db.session.commit()
|
|
|
|
def run_in_indexing_status(self, dataset_document: DatasetDocument):
|
|
"""Run the indexing process when the index_status is indexing."""
|
|
try:
|
|
# get dataset
|
|
dataset = Dataset.query.filter_by(id=dataset_document.dataset_id).first()
|
|
|
|
if not dataset:
|
|
raise ValueError("no dataset found")
|
|
|
|
# get exist document_segment list and delete
|
|
document_segments = DocumentSegment.query.filter_by(
|
|
dataset_id=dataset.id, document_id=dataset_document.id
|
|
).all()
|
|
|
|
documents = []
|
|
if document_segments:
|
|
for document_segment in document_segments:
|
|
# transform segment to node
|
|
if document_segment.status != "completed":
|
|
document = Document(
|
|
page_content=document_segment.content,
|
|
metadata={
|
|
"doc_id": document_segment.index_node_id,
|
|
"doc_hash": document_segment.index_node_hash,
|
|
"document_id": document_segment.document_id,
|
|
"dataset_id": document_segment.dataset_id,
|
|
},
|
|
)
|
|
if dataset_document.doc_form == IndexType.PARENT_CHILD_INDEX:
|
|
child_chunks = document_segment.child_chunks
|
|
if child_chunks:
|
|
child_documents = []
|
|
for child_chunk in child_chunks:
|
|
child_document = ChildDocument(
|
|
page_content=child_chunk.content,
|
|
metadata={
|
|
"doc_id": child_chunk.index_node_id,
|
|
"doc_hash": child_chunk.index_node_hash,
|
|
"document_id": document_segment.document_id,
|
|
"dataset_id": document_segment.dataset_id,
|
|
},
|
|
)
|
|
child_documents.append(child_document)
|
|
document.children = child_documents
|
|
documents.append(document)
|
|
|
|
# build index
|
|
# get the process rule
|
|
processing_rule = (
|
|
db.session.query(DatasetProcessRule)
|
|
.filter(DatasetProcessRule.id == dataset_document.dataset_process_rule_id)
|
|
.first()
|
|
)
|
|
|
|
index_type = dataset_document.doc_form
|
|
index_processor = IndexProcessorFactory(index_type).init_index_processor()
|
|
self._load(
|
|
index_processor=index_processor, dataset=dataset, dataset_document=dataset_document, documents=documents
|
|
)
|
|
except DocumentIsPausedError:
|
|
raise DocumentIsPausedError("Document paused, document id: {}".format(dataset_document.id))
|
|
except ProviderTokenNotInitError as e:
|
|
dataset_document.indexing_status = "error"
|
|
dataset_document.error = str(e.description)
|
|
dataset_document.stopped_at = datetime.datetime.now(datetime.UTC).replace(tzinfo=None)
|
|
db.session.commit()
|
|
except Exception as e:
|
|
logging.exception("consume document failed")
|
|
dataset_document.indexing_status = "error"
|
|
dataset_document.error = str(e)
|
|
dataset_document.stopped_at = datetime.datetime.now(datetime.UTC).replace(tzinfo=None)
|
|
db.session.commit()
|
|
|
|
def indexing_estimate(
|
|
self,
|
|
tenant_id: str,
|
|
extract_settings: list[ExtractSetting],
|
|
tmp_processing_rule: dict,
|
|
doc_form: Optional[str] = None,
|
|
doc_language: str = "English",
|
|
dataset_id: Optional[str] = None,
|
|
indexing_technique: str = "economy",
|
|
) -> IndexingEstimate:
|
|
"""
|
|
Estimate the indexing for the document.
|
|
"""
|
|
# check document limit
|
|
features = FeatureService.get_features(tenant_id)
|
|
if features.billing.enabled:
|
|
count = len(extract_settings)
|
|
batch_upload_limit = dify_config.BATCH_UPLOAD_LIMIT
|
|
if count > batch_upload_limit:
|
|
raise ValueError(f"You have reached the batch upload limit of {batch_upload_limit}.")
|
|
|
|
embedding_model_instance = None
|
|
if dataset_id:
|
|
dataset = Dataset.query.filter_by(id=dataset_id).first()
|
|
if not dataset:
|
|
raise ValueError("Dataset not found.")
|
|
if dataset.indexing_technique == "high_quality" or indexing_technique == "high_quality":
|
|
if dataset.embedding_model_provider:
|
|
embedding_model_instance = self.model_manager.get_model_instance(
|
|
tenant_id=tenant_id,
|
|
provider=dataset.embedding_model_provider,
|
|
model_type=ModelType.TEXT_EMBEDDING,
|
|
model=dataset.embedding_model,
|
|
)
|
|
else:
|
|
embedding_model_instance = self.model_manager.get_default_model_instance(
|
|
tenant_id=tenant_id,
|
|
model_type=ModelType.TEXT_EMBEDDING,
|
|
)
|
|
else:
|
|
if indexing_technique == "high_quality":
|
|
embedding_model_instance = self.model_manager.get_default_model_instance(
|
|
tenant_id=tenant_id,
|
|
model_type=ModelType.TEXT_EMBEDDING,
|
|
)
|
|
preview_texts = [] # type: ignore
|
|
|
|
total_segments = 0
|
|
index_type = doc_form
|
|
index_processor = IndexProcessorFactory(index_type).init_index_processor()
|
|
for extract_setting in extract_settings:
|
|
# extract
|
|
processing_rule = DatasetProcessRule(
|
|
mode=tmp_processing_rule["mode"], rules=json.dumps(tmp_processing_rule["rules"])
|
|
)
|
|
text_docs = index_processor.extract(extract_setting, process_rule_mode=tmp_processing_rule["mode"])
|
|
documents = index_processor.transform(
|
|
text_docs,
|
|
embedding_model_instance=embedding_model_instance,
|
|
process_rule=processing_rule.to_dict(),
|
|
tenant_id=current_user.current_tenant_id,
|
|
doc_language=doc_language,
|
|
preview=True,
|
|
)
|
|
total_segments += len(documents)
|
|
for document in documents:
|
|
if len(preview_texts) < 10:
|
|
if doc_form and doc_form == "qa_model":
|
|
preview_detail = QAPreviewDetail(
|
|
question=document.page_content, answer=document.metadata.get("answer") or ""
|
|
)
|
|
preview_texts.append(preview_detail)
|
|
else:
|
|
preview_detail = PreviewDetail(content=document.page_content) # type: ignore
|
|
if document.children:
|
|
preview_detail.child_chunks = [child.page_content for child in document.children] # type: ignore
|
|
preview_texts.append(preview_detail)
|
|
|
|
# delete image files and related db records
|
|
image_upload_file_ids = get_image_upload_file_ids(document.page_content)
|
|
for upload_file_id in image_upload_file_ids:
|
|
image_file = db.session.query(UploadFile).filter(UploadFile.id == upload_file_id).first()
|
|
try:
|
|
if image_file:
|
|
storage.delete(image_file.key)
|
|
except Exception:
|
|
logging.exception(
|
|
"Delete image_files failed while indexing_estimate, \
|
|
image_upload_file_is: {}".format(upload_file_id)
|
|
)
|
|
db.session.delete(image_file)
|
|
|
|
if doc_form and doc_form == "qa_model":
|
|
return IndexingEstimate(total_segments=total_segments * 20, qa_preview=preview_texts, preview=[])
|
|
return IndexingEstimate(total_segments=total_segments, preview=preview_texts) # type: ignore
|
|
|
|
def _extract(
|
|
self, index_processor: BaseIndexProcessor, dataset_document: DatasetDocument, process_rule: dict
|
|
) -> list[Document]:
|
|
# load file
|
|
if dataset_document.data_source_type not in {"upload_file", "notion_import", "website_crawl"}:
|
|
return []
|
|
|
|
data_source_info = dataset_document.data_source_info_dict
|
|
text_docs = []
|
|
if dataset_document.data_source_type == "upload_file":
|
|
if not data_source_info or "upload_file_id" not in data_source_info:
|
|
raise ValueError("no upload file found")
|
|
|
|
file_detail = (
|
|
db.session.query(UploadFile).filter(UploadFile.id == data_source_info["upload_file_id"]).one_or_none()
|
|
)
|
|
|
|
if file_detail:
|
|
extract_setting = ExtractSetting(
|
|
datasource_type="upload_file", upload_file=file_detail, document_model=dataset_document.doc_form
|
|
)
|
|
text_docs = index_processor.extract(extract_setting, process_rule_mode=process_rule["mode"])
|
|
elif dataset_document.data_source_type == "notion_import":
|
|
if (
|
|
not data_source_info
|
|
or "notion_workspace_id" not in data_source_info
|
|
or "notion_page_id" not in data_source_info
|
|
):
|
|
raise ValueError("no notion import info found")
|
|
extract_setting = ExtractSetting(
|
|
datasource_type="notion_import",
|
|
notion_info={
|
|
"notion_workspace_id": data_source_info["notion_workspace_id"],
|
|
"notion_obj_id": data_source_info["notion_page_id"],
|
|
"notion_page_type": data_source_info["type"],
|
|
"document": dataset_document,
|
|
"tenant_id": dataset_document.tenant_id,
|
|
},
|
|
document_model=dataset_document.doc_form,
|
|
)
|
|
text_docs = index_processor.extract(extract_setting, process_rule_mode=process_rule["mode"])
|
|
elif dataset_document.data_source_type == "website_crawl":
|
|
if (
|
|
not data_source_info
|
|
or "provider" not in data_source_info
|
|
or "url" not in data_source_info
|
|
or "job_id" not in data_source_info
|
|
):
|
|
raise ValueError("no website import info found")
|
|
extract_setting = ExtractSetting(
|
|
datasource_type="website_crawl",
|
|
website_info={
|
|
"provider": data_source_info["provider"],
|
|
"job_id": data_source_info["job_id"],
|
|
"tenant_id": dataset_document.tenant_id,
|
|
"url": data_source_info["url"],
|
|
"mode": data_source_info["mode"],
|
|
"only_main_content": data_source_info["only_main_content"],
|
|
},
|
|
document_model=dataset_document.doc_form,
|
|
)
|
|
text_docs = index_processor.extract(extract_setting, process_rule_mode=process_rule["mode"])
|
|
# update document status to splitting
|
|
self._update_document_index_status(
|
|
document_id=dataset_document.id,
|
|
after_indexing_status="splitting",
|
|
extra_update_params={
|
|
DatasetDocument.word_count: sum(len(text_doc.page_content) for text_doc in text_docs),
|
|
DatasetDocument.parsing_completed_at: datetime.datetime.now(datetime.UTC).replace(tzinfo=None),
|
|
},
|
|
)
|
|
|
|
# replace doc id to document model id
|
|
text_docs = cast(list[Document], text_docs)
|
|
for text_doc in text_docs:
|
|
if text_doc.metadata is not None:
|
|
text_doc.metadata["document_id"] = dataset_document.id
|
|
text_doc.metadata["dataset_id"] = dataset_document.dataset_id
|
|
|
|
return text_docs
|
|
|
|
@staticmethod
|
|
def filter_string(text):
|
|
text = re.sub(r"<\|", "<", text)
|
|
text = re.sub(r"\|>", ">", text)
|
|
text = re.sub(r"[\x00-\x08\x0B\x0C\x0E-\x1F\x7F\xEF\xBF\xBE]", "", text)
|
|
# Unicode U+FFFE
|
|
text = re.sub("\ufffe", "", text)
|
|
return text
|
|
|
|
@staticmethod
|
|
def _get_splitter(
|
|
processing_rule_mode: str,
|
|
max_tokens: int,
|
|
chunk_overlap: int,
|
|
separator: str,
|
|
embedding_model_instance: Optional[ModelInstance],
|
|
) -> TextSplitter:
|
|
"""
|
|
Get the NodeParser object according to the processing rule.
|
|
"""
|
|
if processing_rule_mode in ["custom", "hierarchical"]:
|
|
# The user-defined segmentation rule
|
|
max_segmentation_tokens_length = dify_config.INDEXING_MAX_SEGMENTATION_TOKENS_LENGTH
|
|
if max_tokens < 50 or max_tokens > max_segmentation_tokens_length:
|
|
raise ValueError(f"Custom segment length should be between 50 and {max_segmentation_tokens_length}.")
|
|
|
|
if separator:
|
|
separator = separator.replace("\\n", "\n")
|
|
|
|
character_splitter = FixedRecursiveCharacterTextSplitter.from_encoder(
|
|
chunk_size=max_tokens,
|
|
chunk_overlap=chunk_overlap,
|
|
fixed_separator=separator,
|
|
separators=["\n\n", "。", ". ", " ", ""],
|
|
embedding_model_instance=embedding_model_instance,
|
|
)
|
|
else:
|
|
# Automatic segmentation
|
|
automatic_rules: dict[str, Any] = dict(DatasetProcessRule.AUTOMATIC_RULES["segmentation"])
|
|
character_splitter = EnhanceRecursiveCharacterTextSplitter.from_encoder(
|
|
chunk_size=automatic_rules["max_tokens"],
|
|
chunk_overlap=automatic_rules["chunk_overlap"],
|
|
separators=["\n\n", "。", ". ", " ", ""],
|
|
embedding_model_instance=embedding_model_instance,
|
|
)
|
|
|
|
return character_splitter # type: ignore
|
|
|
|
def _split_to_documents_for_estimate(
|
|
self, text_docs: list[Document], splitter: TextSplitter, processing_rule: DatasetProcessRule
|
|
) -> list[Document]:
|
|
"""
|
|
Split the text documents into nodes.
|
|
"""
|
|
all_documents: list[Document] = []
|
|
for text_doc in text_docs:
|
|
# document clean
|
|
document_text = self._document_clean(text_doc.page_content, processing_rule)
|
|
text_doc.page_content = document_text
|
|
|
|
# parse document to nodes
|
|
documents = splitter.split_documents([text_doc])
|
|
|
|
split_documents = []
|
|
for document in documents:
|
|
if document.page_content is None or not document.page_content.strip():
|
|
continue
|
|
if document.metadata is not None:
|
|
doc_id = str(uuid.uuid4())
|
|
hash = helper.generate_text_hash(document.page_content)
|
|
document.metadata["doc_id"] = doc_id
|
|
document.metadata["doc_hash"] = hash
|
|
|
|
split_documents.append(document)
|
|
|
|
all_documents.extend(split_documents)
|
|
|
|
return all_documents
|
|
|
|
@staticmethod
|
|
def _document_clean(text: str, processing_rule: DatasetProcessRule) -> str:
|
|
"""
|
|
Clean the document text according to the processing rules.
|
|
"""
|
|
if processing_rule.mode == "automatic":
|
|
rules = DatasetProcessRule.AUTOMATIC_RULES
|
|
else:
|
|
rules = json.loads(processing_rule.rules) if processing_rule.rules else {}
|
|
document_text = CleanProcessor.clean(text, {"rules": rules})
|
|
|
|
return document_text
|
|
|
|
@staticmethod
|
|
def format_split_text(text: str) -> list[QAPreviewDetail]:
|
|
regex = r"Q\d+:\s*(.*?)\s*A\d+:\s*([\s\S]*?)(?=Q\d+:|$)"
|
|
matches = re.findall(regex, text, re.UNICODE)
|
|
|
|
return [QAPreviewDetail(question=q, answer=re.sub(r"\n\s*", "\n", a.strip())) for q, a in matches if q and a]
|
|
|
|
def _load(
|
|
self,
|
|
index_processor: BaseIndexProcessor,
|
|
dataset: Dataset,
|
|
dataset_document: DatasetDocument,
|
|
documents: list[Document],
|
|
) -> None:
|
|
"""
|
|
insert index and update document/segment status to completed
|
|
"""
|
|
|
|
embedding_model_instance = None
|
|
if dataset.indexing_technique == "high_quality":
|
|
embedding_model_instance = self.model_manager.get_model_instance(
|
|
tenant_id=dataset.tenant_id,
|
|
provider=dataset.embedding_model_provider,
|
|
model_type=ModelType.TEXT_EMBEDDING,
|
|
model=dataset.embedding_model,
|
|
)
|
|
|
|
# chunk nodes by chunk size
|
|
indexing_start_at = time.perf_counter()
|
|
tokens = 0
|
|
if dataset_document.doc_form != IndexType.PARENT_CHILD_INDEX:
|
|
# create keyword index
|
|
create_keyword_thread = threading.Thread(
|
|
target=self._process_keyword_index,
|
|
args=(current_app._get_current_object(), dataset.id, dataset_document.id, documents), # type: ignore
|
|
)
|
|
create_keyword_thread.start()
|
|
|
|
max_workers = 10
|
|
if dataset.indexing_technique == "high_quality":
|
|
with concurrent.futures.ThreadPoolExecutor(max_workers=max_workers) as executor:
|
|
futures = []
|
|
|
|
# Distribute documents into multiple groups based on the hash values of page_content
|
|
# This is done to prevent multiple threads from processing the same document,
|
|
# Thereby avoiding potential database insertion deadlocks
|
|
document_groups: list[list[Document]] = [[] for _ in range(max_workers)]
|
|
for document in documents:
|
|
hash = helper.generate_text_hash(document.page_content)
|
|
group_index = int(hash, 16) % max_workers
|
|
document_groups[group_index].append(document)
|
|
for chunk_documents in document_groups:
|
|
if len(chunk_documents) == 0:
|
|
continue
|
|
futures.append(
|
|
executor.submit(
|
|
self._process_chunk,
|
|
current_app._get_current_object(), # type: ignore
|
|
index_processor,
|
|
chunk_documents,
|
|
dataset,
|
|
dataset_document,
|
|
embedding_model_instance,
|
|
)
|
|
)
|
|
|
|
for future in futures:
|
|
tokens += future.result()
|
|
if dataset_document.doc_form != IndexType.PARENT_CHILD_INDEX:
|
|
create_keyword_thread.join()
|
|
indexing_end_at = time.perf_counter()
|
|
|
|
# update document status to completed
|
|
self._update_document_index_status(
|
|
document_id=dataset_document.id,
|
|
after_indexing_status="completed",
|
|
extra_update_params={
|
|
DatasetDocument.tokens: tokens,
|
|
DatasetDocument.completed_at: datetime.datetime.now(datetime.UTC).replace(tzinfo=None),
|
|
DatasetDocument.indexing_latency: indexing_end_at - indexing_start_at,
|
|
DatasetDocument.error: None,
|
|
},
|
|
)
|
|
|
|
@staticmethod
|
|
def _process_keyword_index(flask_app, dataset_id, document_id, documents):
|
|
with flask_app.app_context():
|
|
dataset = Dataset.query.filter_by(id=dataset_id).first()
|
|
if not dataset:
|
|
raise ValueError("no dataset found")
|
|
keyword = Keyword(dataset)
|
|
keyword.create(documents)
|
|
if dataset.indexing_technique != "high_quality":
|
|
document_ids = [document.metadata["doc_id"] for document in documents]
|
|
db.session.query(DocumentSegment).filter(
|
|
DocumentSegment.document_id == document_id,
|
|
DocumentSegment.dataset_id == dataset_id,
|
|
DocumentSegment.index_node_id.in_(document_ids),
|
|
DocumentSegment.status == "indexing",
|
|
).update(
|
|
{
|
|
DocumentSegment.status: "completed",
|
|
DocumentSegment.enabled: True,
|
|
DocumentSegment.completed_at: datetime.datetime.now(datetime.UTC).replace(tzinfo=None),
|
|
}
|
|
)
|
|
|
|
db.session.commit()
|
|
|
|
def _process_chunk(
|
|
self, flask_app, index_processor, chunk_documents, dataset, dataset_document, embedding_model_instance
|
|
):
|
|
with flask_app.app_context():
|
|
# check document is paused
|
|
self._check_document_paused_status(dataset_document.id)
|
|
|
|
tokens = 0
|
|
if embedding_model_instance:
|
|
page_content_list = [document.page_content for document in chunk_documents]
|
|
tokens += sum(embedding_model_instance.get_text_embedding_num_tokens(page_content_list))
|
|
|
|
# load index
|
|
index_processor.load(dataset, chunk_documents, with_keywords=False)
|
|
|
|
document_ids = [document.metadata["doc_id"] for document in chunk_documents]
|
|
db.session.query(DocumentSegment).filter(
|
|
DocumentSegment.document_id == dataset_document.id,
|
|
DocumentSegment.dataset_id == dataset.id,
|
|
DocumentSegment.index_node_id.in_(document_ids),
|
|
DocumentSegment.status == "indexing",
|
|
).update(
|
|
{
|
|
DocumentSegment.status: "completed",
|
|
DocumentSegment.enabled: True,
|
|
DocumentSegment.completed_at: datetime.datetime.now(datetime.UTC).replace(tzinfo=None),
|
|
}
|
|
)
|
|
|
|
db.session.commit()
|
|
|
|
return tokens
|
|
|
|
@staticmethod
|
|
def _check_document_paused_status(document_id: str):
|
|
indexing_cache_key = "document_{}_is_paused".format(document_id)
|
|
result = redis_client.get(indexing_cache_key)
|
|
if result:
|
|
raise DocumentIsPausedError()
|
|
|
|
@staticmethod
|
|
def _update_document_index_status(
|
|
document_id: str, after_indexing_status: str, extra_update_params: Optional[dict] = None
|
|
) -> None:
|
|
"""
|
|
Update the document indexing status.
|
|
"""
|
|
count = DatasetDocument.query.filter_by(id=document_id, is_paused=True).count()
|
|
if count > 0:
|
|
raise DocumentIsPausedError()
|
|
document = DatasetDocument.query.filter_by(id=document_id).first()
|
|
if not document:
|
|
raise DocumentIsDeletedPausedError()
|
|
|
|
update_params = {DatasetDocument.indexing_status: after_indexing_status}
|
|
|
|
if extra_update_params:
|
|
update_params.update(extra_update_params)
|
|
|
|
DatasetDocument.query.filter_by(id=document_id).update(update_params)
|
|
db.session.commit()
|
|
|
|
@staticmethod
|
|
def _update_segments_by_document(dataset_document_id: str, update_params: dict) -> None:
|
|
"""
|
|
Update the document segment by document id.
|
|
"""
|
|
DocumentSegment.query.filter_by(document_id=dataset_document_id).update(update_params)
|
|
db.session.commit()
|
|
|
|
def _transform(
|
|
self,
|
|
index_processor: BaseIndexProcessor,
|
|
dataset: Dataset,
|
|
text_docs: list[Document],
|
|
doc_language: str,
|
|
process_rule: dict,
|
|
) -> list[Document]:
|
|
# get embedding model instance
|
|
embedding_model_instance = None
|
|
if dataset.indexing_technique == "high_quality":
|
|
if dataset.embedding_model_provider:
|
|
embedding_model_instance = self.model_manager.get_model_instance(
|
|
tenant_id=dataset.tenant_id,
|
|
provider=dataset.embedding_model_provider,
|
|
model_type=ModelType.TEXT_EMBEDDING,
|
|
model=dataset.embedding_model,
|
|
)
|
|
else:
|
|
embedding_model_instance = self.model_manager.get_default_model_instance(
|
|
tenant_id=dataset.tenant_id,
|
|
model_type=ModelType.TEXT_EMBEDDING,
|
|
)
|
|
|
|
documents = index_processor.transform(
|
|
text_docs,
|
|
embedding_model_instance=embedding_model_instance,
|
|
process_rule=process_rule,
|
|
tenant_id=dataset.tenant_id,
|
|
doc_language=doc_language,
|
|
)
|
|
|
|
return documents
|
|
|
|
def _load_segments(self, dataset, dataset_document, documents):
|
|
# save node to document segment
|
|
doc_store = DatasetDocumentStore(
|
|
dataset=dataset, user_id=dataset_document.created_by, document_id=dataset_document.id
|
|
)
|
|
|
|
# add document segments
|
|
doc_store.add_documents(docs=documents, save_child=dataset_document.doc_form == IndexType.PARENT_CHILD_INDEX)
|
|
|
|
# update document status to indexing
|
|
cur_time = datetime.datetime.now(datetime.UTC).replace(tzinfo=None)
|
|
self._update_document_index_status(
|
|
document_id=dataset_document.id,
|
|
after_indexing_status="indexing",
|
|
extra_update_params={
|
|
DatasetDocument.cleaning_completed_at: cur_time,
|
|
DatasetDocument.splitting_completed_at: cur_time,
|
|
},
|
|
)
|
|
|
|
# update segment status to indexing
|
|
self._update_segments_by_document(
|
|
dataset_document_id=dataset_document.id,
|
|
update_params={
|
|
DocumentSegment.status: "indexing",
|
|
DocumentSegment.indexing_at: datetime.datetime.now(datetime.UTC).replace(tzinfo=None),
|
|
},
|
|
)
|
|
pass
|
|
|
|
|
|
class DocumentIsPausedError(Exception):
|
|
pass
|
|
|
|
|
|
class DocumentIsDeletedPausedError(Exception):
|
|
pass
|