mirror of
https://git.mirrors.martin98.com/https://github.com/infiniflow/ragflow.git
synced 2025-04-22 14:10:01 +08:00
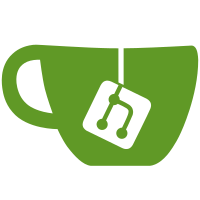
This commit refactors the deep research module (deep_research.py), with the following major improvements: The complex thinking and retrieval logic has been broken down into multiple independent private methods, enhancing code readability and maintainability. Static methods and class methods have been introduced to simplify the logic for tag processing. The search and reasoning processes have been optimized, increasing the modularity of the code. The flexibility of information retrieval and processing has been improved. The refactored code structure is now clearer, making it easier to understand and extend the functionality of the deep research module. ### What problem does this PR solve? increase the modularity of the code ### Type of change - [x] Refactoring Co-authored-by: wenju.li <wenju.li@deepctr.cn>
224 lines
10 KiB
Python
224 lines
10 KiB
Python
#
|
|
# Copyright 2024 The InfiniFlow Authors. All Rights Reserved.
|
|
#
|
|
# Licensed under the Apache License, Version 2.0 (the "License");
|
|
# you may not use this file except in compliance with the License.
|
|
# You may obtain a copy of the License at
|
|
#
|
|
# http://www.apache.org/licenses/LICENSE-2.0
|
|
#
|
|
# Unless required by applicable law or agreed to in writing, software
|
|
# distributed under the License is distributed on an "AS IS" BASIS,
|
|
# WITHOUT WARRANTIES OR CONDITIONS OF ANY KIND, either express or implied.
|
|
# See the License for the specific language governing permissions and
|
|
# limitations under the License.
|
|
#
|
|
import logging
|
|
import re
|
|
from functools import partial
|
|
from agentic_reasoning.prompts import BEGIN_SEARCH_QUERY, BEGIN_SEARCH_RESULT, END_SEARCH_RESULT, MAX_SEARCH_LIMIT, \
|
|
END_SEARCH_QUERY, REASON_PROMPT, RELEVANT_EXTRACTION_PROMPT
|
|
from api.db.services.llm_service import LLMBundle
|
|
from rag.nlp import extract_between
|
|
from rag.prompts import kb_prompt
|
|
from rag.utils.tavily_conn import Tavily
|
|
|
|
|
|
class DeepResearcher:
|
|
def __init__(self,
|
|
chat_mdl: LLMBundle,
|
|
prompt_config: dict,
|
|
kb_retrieve: partial = None,
|
|
kg_retrieve: partial = None
|
|
):
|
|
self.chat_mdl = chat_mdl
|
|
self.prompt_config = prompt_config
|
|
self._kb_retrieve = kb_retrieve
|
|
self._kg_retrieve = kg_retrieve
|
|
|
|
@staticmethod
|
|
def _remove_query_tags(text):
|
|
"""Remove query tags from text"""
|
|
pattern = re.escape(BEGIN_SEARCH_QUERY) + r"(.*?)" + re.escape(END_SEARCH_QUERY)
|
|
return re.sub(pattern, "", text)
|
|
|
|
@staticmethod
|
|
def _remove_result_tags(text):
|
|
"""Remove result tags from text"""
|
|
pattern = re.escape(BEGIN_SEARCH_RESULT) + r"(.*?)" + re.escape(END_SEARCH_RESULT)
|
|
return re.sub(pattern, "", text)
|
|
|
|
def _generate_reasoning(self, msg_history):
|
|
"""Generate reasoning steps"""
|
|
query_think = ""
|
|
if msg_history[-1]["role"] != "user":
|
|
msg_history.append({"role": "user", "content": "Continues reasoning with the new information.\n"})
|
|
else:
|
|
msg_history[-1]["content"] += "\n\nContinues reasoning with the new information.\n"
|
|
|
|
for ans in self.chat_mdl.chat_streamly(REASON_PROMPT, msg_history, {"temperature": 0.7}):
|
|
ans = re.sub(r"<think>.*</think>", "", ans, flags=re.DOTALL)
|
|
if not ans:
|
|
continue
|
|
query_think = ans
|
|
yield query_think
|
|
return query_think
|
|
|
|
def _extract_search_queries(self, query_think, question, step_index):
|
|
"""Extract search queries from thinking"""
|
|
queries = extract_between(query_think, BEGIN_SEARCH_QUERY, END_SEARCH_QUERY)
|
|
if not queries and step_index == 0:
|
|
# If this is the first step and no queries are found, use the original question as the query
|
|
queries = [question]
|
|
return queries
|
|
|
|
def _truncate_previous_reasoning(self, all_reasoning_steps):
|
|
"""Truncate previous reasoning steps to maintain a reasonable length"""
|
|
truncated_prev_reasoning = ""
|
|
for i, step in enumerate(all_reasoning_steps):
|
|
truncated_prev_reasoning += f"Step {i + 1}: {step}\n\n"
|
|
|
|
prev_steps = truncated_prev_reasoning.split('\n\n')
|
|
if len(prev_steps) <= 5:
|
|
truncated_prev_reasoning = '\n\n'.join(prev_steps)
|
|
else:
|
|
truncated_prev_reasoning = ''
|
|
for i, step in enumerate(prev_steps):
|
|
if i == 0 or i >= len(prev_steps) - 4 or BEGIN_SEARCH_QUERY in step or BEGIN_SEARCH_RESULT in step:
|
|
truncated_prev_reasoning += step + '\n\n'
|
|
else:
|
|
if truncated_prev_reasoning[-len('\n\n...\n\n'):] != '\n\n...\n\n':
|
|
truncated_prev_reasoning += '...\n\n'
|
|
|
|
return truncated_prev_reasoning.strip('\n')
|
|
|
|
def _retrieve_information(self, search_query):
|
|
"""Retrieve information from different sources"""
|
|
# 1. Knowledge base retrieval
|
|
kbinfos = self._kb_retrieve(question=search_query) if self._kb_retrieve else {"chunks": [], "doc_aggs": []}
|
|
|
|
# 2. Web retrieval (if Tavily API is configured)
|
|
if self.prompt_config.get("tavily_api_key"):
|
|
tav = Tavily(self.prompt_config["tavily_api_key"])
|
|
tav_res = tav.retrieve_chunks(search_query)
|
|
kbinfos["chunks"].extend(tav_res["chunks"])
|
|
kbinfos["doc_aggs"].extend(tav_res["doc_aggs"])
|
|
|
|
# 3. Knowledge graph retrieval (if configured)
|
|
if self.prompt_config.get("use_kg") and self._kg_retrieve:
|
|
ck = self._kg_retrieve(question=search_query)
|
|
if ck["content_with_weight"]:
|
|
kbinfos["chunks"].insert(0, ck)
|
|
|
|
return kbinfos
|
|
|
|
def _update_chunk_info(self, chunk_info, kbinfos):
|
|
"""Update chunk information for citations"""
|
|
if not chunk_info["chunks"]:
|
|
# If this is the first retrieval, use the retrieval results directly
|
|
for k in chunk_info.keys():
|
|
chunk_info[k] = kbinfos[k]
|
|
else:
|
|
# Merge newly retrieved information, avoiding duplicates
|
|
cids = [c["chunk_id"] for c in chunk_info["chunks"]]
|
|
for c in kbinfos["chunks"]:
|
|
if c["chunk_id"] not in cids:
|
|
chunk_info["chunks"].append(c)
|
|
|
|
dids = [d["doc_id"] for d in chunk_info["doc_aggs"]]
|
|
for d in kbinfos["doc_aggs"]:
|
|
if d["doc_id"] not in dids:
|
|
chunk_info["doc_aggs"].append(d)
|
|
|
|
def _extract_relevant_info(self, truncated_prev_reasoning, search_query, kbinfos):
|
|
"""Extract and summarize relevant information"""
|
|
summary_think = ""
|
|
for ans in self.chat_mdl.chat_streamly(
|
|
RELEVANT_EXTRACTION_PROMPT.format(
|
|
prev_reasoning=truncated_prev_reasoning,
|
|
search_query=search_query,
|
|
document="\n".join(kb_prompt(kbinfos, 4096))
|
|
),
|
|
[{"role": "user",
|
|
"content": f'Now you should analyze each web page and find helpful information based on the current search query "{search_query}" and previous reasoning steps.'}],
|
|
{"temperature": 0.7}):
|
|
ans = re.sub(r"<think>.*</think>", "", ans, flags=re.DOTALL)
|
|
if not ans:
|
|
continue
|
|
summary_think = ans
|
|
yield summary_think
|
|
|
|
return summary_think
|
|
|
|
def thinking(self, chunk_info: dict, question: str):
|
|
executed_search_queries = []
|
|
msg_history = [{"role": "user", "content": f'Question:\"{question}\"\n'}]
|
|
all_reasoning_steps = []
|
|
think = "<think>"
|
|
|
|
for step_index in range(MAX_SEARCH_LIMIT + 1):
|
|
# Check if the maximum search limit has been reached
|
|
if step_index == MAX_SEARCH_LIMIT - 1:
|
|
summary_think = f"\n{BEGIN_SEARCH_RESULT}\nThe maximum search limit is exceeded. You are not allowed to search.\n{END_SEARCH_RESULT}\n"
|
|
yield {"answer": think + summary_think + "</think>", "reference": {}, "audio_binary": None}
|
|
all_reasoning_steps.append(summary_think)
|
|
msg_history.append({"role": "assistant", "content": summary_think})
|
|
break
|
|
|
|
# Step 1: Generate reasoning
|
|
query_think = ""
|
|
for ans in self._generate_reasoning(msg_history):
|
|
query_think = ans
|
|
yield {"answer": think + self._remove_query_tags(query_think) + "</think>", "reference": {}, "audio_binary": None}
|
|
|
|
think += self._remove_query_tags(query_think)
|
|
all_reasoning_steps.append(query_think)
|
|
|
|
# Step 2: Extract search queries
|
|
queries = self._extract_search_queries(query_think, question, step_index)
|
|
if not queries and step_index > 0:
|
|
# If not the first step and no queries, end the search process
|
|
break
|
|
|
|
# Process each search query
|
|
for search_query in queries:
|
|
logging.info(f"[THINK]Query: {step_index}. {search_query}")
|
|
msg_history.append({"role": "assistant", "content": search_query})
|
|
think += f"\n\n> {step_index + 1}. {search_query}\n\n"
|
|
yield {"answer": think + "</think>", "reference": {}, "audio_binary": None}
|
|
|
|
# Check if the query has already been executed
|
|
if search_query in executed_search_queries:
|
|
summary_think = f"\n{BEGIN_SEARCH_RESULT}\nYou have searched this query. Please refer to previous results.\n{END_SEARCH_RESULT}\n"
|
|
yield {"answer": think + summary_think + "</think>", "reference": {}, "audio_binary": None}
|
|
all_reasoning_steps.append(summary_think)
|
|
msg_history.append({"role": "user", "content": summary_think})
|
|
think += summary_think
|
|
continue
|
|
|
|
executed_search_queries.append(search_query)
|
|
|
|
# Step 3: Truncate previous reasoning steps
|
|
truncated_prev_reasoning = self._truncate_previous_reasoning(all_reasoning_steps)
|
|
|
|
# Step 4: Retrieve information
|
|
kbinfos = self._retrieve_information(search_query)
|
|
|
|
# Step 5: Update chunk information
|
|
self._update_chunk_info(chunk_info, kbinfos)
|
|
|
|
# Step 6: Extract relevant information
|
|
think += "\n\n"
|
|
summary_think = ""
|
|
for ans in self._extract_relevant_info(truncated_prev_reasoning, search_query, kbinfos):
|
|
summary_think = ans
|
|
yield {"answer": think + self._remove_result_tags(summary_think) + "</think>", "reference": {}, "audio_binary": None}
|
|
|
|
all_reasoning_steps.append(summary_think)
|
|
msg_history.append(
|
|
{"role": "user", "content": f"\n\n{BEGIN_SEARCH_RESULT}{summary_think}{END_SEARCH_RESULT}\n\n"})
|
|
think += self._remove_result_tags(summary_think)
|
|
logging.info(f"[THINK]Summary: {step_index}. {summary_think}")
|
|
|
|
yield think + "</think>"
|