mirror of
https://git.mirrors.martin98.com/https://github.com/infiniflow/ragflow.git
synced 2025-07-15 08:21:48 +08:00
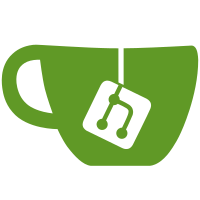
## Problem Description Multiple files in the RAGFlow project contain closure trap issues when using lambda functions with `trio.open_nursery()`. This problem causes concurrent tasks created in loops to reference the same variable, resulting in all tasks processing the same data (the data from the last iteration) rather than each task processing its corresponding data from the loop. ## Issue Details When using a `lambda` to create a closure function and passing it to `nursery.start_soon()` within a loop, the lambda function captures a reference to the loop variable rather than its value. For example: ```python # Problematic code async with trio.open_nursery() as nursery: for d in docs: nursery.start_soon(lambda: doc_keyword_extraction(chat_mdl, d, topn)) ``` In this pattern, when concurrent tasks begin execution, `d` has already become the value after the loop ends (typically the last element), causing all tasks to use the same data. ## Fix Solution Changed the way concurrent tasks are created with `nursery.start_soon()` by leveraging Trio's API design to directly pass the function and its arguments separately: ```python # Fixed code async with trio.open_nursery() as nursery: for d in docs: nursery.start_soon(doc_keyword_extraction, chat_mdl, d, topn) ``` This way, each task uses the parameter values at the time of the function call, rather than references captured through closures. ## Fixed Files Fixed closure traps in the following files: 1. `rag/svr/task_executor.py`: 3 fixes, involving document keyword extraction, question generation, and tag processing 2. `rag/raptor.py`: 1 fix, involving document summarization 3. `graphrag/utils.py`: 2 fixes, involving graph node and edge processing 4. `graphrag/entity_resolution.py`: 2 fixes, involving entity resolution and graph node merging 5. `graphrag/general/mind_map_extractor.py`: 2 fixes, involving document processing 6. `graphrag/general/extractor.py`: 3 fixes, involving content processing and graph node/edge merging 7. `graphrag/general/community_reports_extractor.py`: 1 fix, involving community report extraction ## Potential Impact This fix resolves a serious concurrency issue that could have caused: - Data processing errors (processing duplicate data) - Performance degradation (all tasks working on the same data) - Inconsistent results (some data not being processed) After the fix, all concurrent tasks should correctly process their respective data, improving system correctness and reliability.
181 lines
6.3 KiB
Python
181 lines
6.3 KiB
Python
#
|
|
# Copyright 2024 The InfiniFlow Authors. All Rights Reserved.
|
|
#
|
|
# Licensed under the Apache License, Version 2.0 (the "License");
|
|
# you may not use this file except in compliance with the License.
|
|
# You may obtain a copy of the License at
|
|
#
|
|
# http://www.apache.org/licenses/LICENSE-2.0
|
|
#
|
|
# Unless required by applicable law or agreed to in writing, software
|
|
# distributed under the License is distributed on an "AS IS" BASIS,
|
|
# WITHOUT WARRANTIES OR CONDITIONS OF ANY KIND, either express or implied.
|
|
# See the License for the specific language governing permissions and
|
|
# limitations under the License.
|
|
#
|
|
|
|
import logging
|
|
import collections
|
|
import re
|
|
from typing import Any
|
|
from dataclasses import dataclass
|
|
import trio
|
|
|
|
from graphrag.general.extractor import Extractor
|
|
from graphrag.general.mind_map_prompt import MIND_MAP_EXTRACTION_PROMPT
|
|
from graphrag.utils import ErrorHandlerFn, perform_variable_replacements, chat_limiter
|
|
from rag.llm.chat_model import Base as CompletionLLM
|
|
import markdown_to_json
|
|
from functools import reduce
|
|
from rag.utils import num_tokens_from_string
|
|
|
|
|
|
@dataclass
|
|
class MindMapResult:
|
|
"""Unipartite Mind Graph result class definition."""
|
|
output: dict
|
|
|
|
|
|
class MindMapExtractor(Extractor):
|
|
_input_text_key: str
|
|
_mind_map_prompt: str
|
|
_on_error: ErrorHandlerFn
|
|
|
|
def __init__(
|
|
self,
|
|
llm_invoker: CompletionLLM,
|
|
prompt: str | None = None,
|
|
input_text_key: str | None = None,
|
|
on_error: ErrorHandlerFn | None = None,
|
|
):
|
|
"""Init method definition."""
|
|
# TODO: streamline construction
|
|
self._llm = llm_invoker
|
|
self._input_text_key = input_text_key or "input_text"
|
|
self._mind_map_prompt = prompt or MIND_MAP_EXTRACTION_PROMPT
|
|
self._on_error = on_error or (lambda _e, _s, _d: None)
|
|
|
|
def _key(self, k):
|
|
return re.sub(r"\*+", "", k)
|
|
|
|
def _be_children(self, obj: dict, keyset: set):
|
|
if isinstance(obj, str):
|
|
obj = [obj]
|
|
if isinstance(obj, list):
|
|
keyset.update(obj)
|
|
obj = [re.sub(r"\*+", "", i) for i in obj]
|
|
return [{"id": i, "children": []} for i in obj if i]
|
|
arr = []
|
|
for k, v in obj.items():
|
|
k = self._key(k)
|
|
if k and k not in keyset:
|
|
keyset.add(k)
|
|
arr.append(
|
|
{
|
|
"id": k,
|
|
"children": self._be_children(v, keyset)
|
|
}
|
|
)
|
|
return arr
|
|
|
|
async def __call__(
|
|
self, sections: list[str], prompt_variables: dict[str, Any] | None = None
|
|
) -> MindMapResult:
|
|
"""Call method definition."""
|
|
if prompt_variables is None:
|
|
prompt_variables = {}
|
|
|
|
res = []
|
|
token_count = max(self._llm.max_length * 0.8, self._llm.max_length - 512)
|
|
texts = []
|
|
cnt = 0
|
|
async with trio.open_nursery() as nursery:
|
|
for i in range(len(sections)):
|
|
section_cnt = num_tokens_from_string(sections[i])
|
|
if cnt + section_cnt >= token_count and texts:
|
|
nursery.start_soon(self._process_document, "".join(texts), prompt_variables, res)
|
|
texts = []
|
|
cnt = 0
|
|
texts.append(sections[i])
|
|
cnt += section_cnt
|
|
if texts:
|
|
nursery.start_soon(self._process_document, "".join(texts), prompt_variables, res)
|
|
if not res:
|
|
return MindMapResult(output={"id": "root", "children": []})
|
|
merge_json = reduce(self._merge, res)
|
|
if len(merge_json) > 1:
|
|
keys = [re.sub(r"\*+", "", k) for k, v in merge_json.items() if isinstance(v, dict)]
|
|
keyset = set(i for i in keys if i)
|
|
merge_json = {
|
|
"id": "root",
|
|
"children": [
|
|
{
|
|
"id": self._key(k),
|
|
"children": self._be_children(v, keyset)
|
|
}
|
|
for k, v in merge_json.items() if isinstance(v, dict) and self._key(k)
|
|
]
|
|
}
|
|
else:
|
|
k = self._key(list(merge_json.keys())[0])
|
|
merge_json = {"id": k, "children": self._be_children(list(merge_json.items())[0][1], {k})}
|
|
|
|
return MindMapResult(output=merge_json)
|
|
|
|
def _merge(self, d1, d2):
|
|
for k in d1:
|
|
if k in d2:
|
|
if isinstance(d1[k], dict) and isinstance(d2[k], dict):
|
|
self._merge(d1[k], d2[k])
|
|
elif isinstance(d1[k], list) and isinstance(d2[k], list):
|
|
d2[k].extend(d1[k])
|
|
else:
|
|
d2[k] = d1[k]
|
|
else:
|
|
d2[k] = d1[k]
|
|
|
|
return d2
|
|
|
|
def _list_to_kv(self, data):
|
|
for key, value in data.items():
|
|
if isinstance(value, dict):
|
|
self._list_to_kv(value)
|
|
elif isinstance(value, list):
|
|
new_value = {}
|
|
for i in range(len(value)):
|
|
if isinstance(value[i], list) and i > 0:
|
|
new_value[value[i - 1]] = value[i][0]
|
|
data[key] = new_value
|
|
else:
|
|
continue
|
|
return data
|
|
|
|
def _todict(self, layer: collections.OrderedDict):
|
|
to_ret = layer
|
|
if isinstance(layer, collections.OrderedDict):
|
|
to_ret = dict(layer)
|
|
|
|
try:
|
|
for key, value in to_ret.items():
|
|
to_ret[key] = self._todict(value)
|
|
except AttributeError:
|
|
pass
|
|
|
|
return self._list_to_kv(to_ret)
|
|
|
|
async def _process_document(
|
|
self, text: str, prompt_variables: dict[str, str], out_res
|
|
) -> str:
|
|
variables = {
|
|
**prompt_variables,
|
|
self._input_text_key: text,
|
|
}
|
|
text = perform_variable_replacements(self._mind_map_prompt, variables=variables)
|
|
gen_conf = {"temperature": 0.5}
|
|
async with chat_limiter:
|
|
response = await trio.to_thread.run_sync(lambda: self._chat(text, [{"role": "user", "content": "Output:"}], gen_conf))
|
|
response = re.sub(r"```[^\n]*", "", response)
|
|
logging.debug(response)
|
|
logging.debug(self._todict(markdown_to_json.dictify(response)))
|
|
out_res.append(self._todict(markdown_to_json.dictify(response)))
|