mirror of
https://git.mirrors.martin98.com/https://github.com/infiniflow/ragflow.git
synced 2025-07-02 07:35:09 +08:00
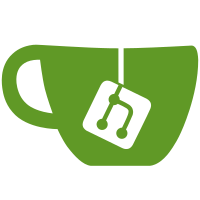
### What problem does this PR solve? Add license statement. ### Type of change - [x] Refactoring Signed-off-by: Jin Hai <haijin.chn@gmail.com>
246 lines
9.4 KiB
Python
246 lines
9.4 KiB
Python
#
|
||
# Copyright 2025 The InfiniFlow Authors. All Rights Reserved.
|
||
#
|
||
# Licensed under the Apache License, Version 2.0 (the "License");
|
||
# you may not use this file except in compliance with the License.
|
||
# You may obtain a copy of the License at
|
||
#
|
||
# http://www.apache.org/licenses/LICENSE-2.0
|
||
#
|
||
# Unless required by applicable law or agreed to in writing, software
|
||
# distributed under the License is distributed on an "AS IS" BASIS,
|
||
# WITHOUT WARRANTIES OR CONDITIONS OF ANY KIND, either express or implied.
|
||
# See the License for the specific language governing permissions and
|
||
# limitations under the License.
|
||
#
|
||
|
||
import os
|
||
import re
|
||
from collections import Counter
|
||
from copy import deepcopy
|
||
|
||
import cv2
|
||
import numpy as np
|
||
from huggingface_hub import snapshot_download
|
||
|
||
from api.utils.file_utils import get_project_base_directory
|
||
from deepdoc.vision import Recognizer
|
||
from deepdoc.vision.operators import nms
|
||
|
||
|
||
class LayoutRecognizer(Recognizer):
|
||
labels = [
|
||
"_background_",
|
||
"Text",
|
||
"Title",
|
||
"Figure",
|
||
"Figure caption",
|
||
"Table",
|
||
"Table caption",
|
||
"Header",
|
||
"Footer",
|
||
"Reference",
|
||
"Equation",
|
||
]
|
||
|
||
def __init__(self, domain):
|
||
try:
|
||
model_dir = os.path.join(
|
||
get_project_base_directory(),
|
||
"rag/res/deepdoc")
|
||
super().__init__(self.labels, domain, model_dir)
|
||
except Exception:
|
||
model_dir = snapshot_download(repo_id="InfiniFlow/deepdoc",
|
||
local_dir=os.path.join(get_project_base_directory(), "rag/res/deepdoc"),
|
||
local_dir_use_symlinks=False)
|
||
super().__init__(self.labels, domain, model_dir)
|
||
|
||
self.garbage_layouts = ["footer", "header", "reference"]
|
||
|
||
def __call__(self, image_list, ocr_res, scale_factor=3,
|
||
thr=0.2, batch_size=16, drop=True):
|
||
def __is_garbage(b):
|
||
patt = [r"^•+$", r"(版权归©|免责条款|地址[::])", r"\.{3,}", "^[0-9]{1,2} / ?[0-9]{1,2}$",
|
||
r"^[0-9]{1,2} of [0-9]{1,2}$", "^http://[^ ]{12,}",
|
||
"(资料|数据)来源[::]", "[0-9a-z._-]+@[a-z0-9-]+\\.[a-z]{2,3}",
|
||
"\\(cid *: *[0-9]+ *\\)"
|
||
]
|
||
return any([re.search(p, b["text"]) for p in patt])
|
||
|
||
layouts = super().__call__(image_list, thr, batch_size)
|
||
# save_results(image_list, layouts, self.labels, output_dir='output/', threshold=0.7)
|
||
assert len(image_list) == len(ocr_res)
|
||
# Tag layout type
|
||
boxes = []
|
||
assert len(image_list) == len(layouts)
|
||
garbages = {}
|
||
page_layout = []
|
||
for pn, lts in enumerate(layouts):
|
||
bxs = ocr_res[pn]
|
||
lts = [{"type": b["type"],
|
||
"score": float(b["score"]),
|
||
"x0": b["bbox"][0] / scale_factor, "x1": b["bbox"][2] / scale_factor,
|
||
"top": b["bbox"][1] / scale_factor, "bottom": b["bbox"][-1] / scale_factor,
|
||
"page_number": pn,
|
||
} for b in lts if float(b["score"]) >= 0.4 or b["type"] not in self.garbage_layouts]
|
||
lts = self.sort_Y_firstly(lts, np.mean(
|
||
[lt["bottom"] - lt["top"] for lt in lts]) / 2)
|
||
lts = self.layouts_cleanup(bxs, lts)
|
||
page_layout.append(lts)
|
||
|
||
# Tag layout type, layouts are ready
|
||
def findLayout(ty):
|
||
nonlocal bxs, lts, self
|
||
lts_ = [lt for lt in lts if lt["type"] == ty]
|
||
i = 0
|
||
while i < len(bxs):
|
||
if bxs[i].get("layout_type"):
|
||
i += 1
|
||
continue
|
||
if __is_garbage(bxs[i]):
|
||
bxs.pop(i)
|
||
continue
|
||
|
||
ii = self.find_overlapped_with_threashold(bxs[i], lts_,
|
||
thr=0.4)
|
||
if ii is None: # belong to nothing
|
||
bxs[i]["layout_type"] = ""
|
||
i += 1
|
||
continue
|
||
lts_[ii]["visited"] = True
|
||
keep_feats = [
|
||
lts_[
|
||
ii]["type"] == "footer" and bxs[i]["bottom"] < image_list[pn].size[1] * 0.9 / scale_factor,
|
||
lts_[
|
||
ii]["type"] == "header" and bxs[i]["top"] > image_list[pn].size[1] * 0.1 / scale_factor,
|
||
]
|
||
if drop and lts_[
|
||
ii]["type"] in self.garbage_layouts and not any(keep_feats):
|
||
if lts_[ii]["type"] not in garbages:
|
||
garbages[lts_[ii]["type"]] = []
|
||
garbages[lts_[ii]["type"]].append(bxs[i]["text"])
|
||
bxs.pop(i)
|
||
continue
|
||
|
||
bxs[i]["layoutno"] = f"{ty}-{ii}"
|
||
bxs[i]["layout_type"] = lts_[ii]["type"] if lts_[
|
||
ii]["type"] != "equation" else "figure"
|
||
i += 1
|
||
|
||
for lt in ["footer", "header", "reference", "figure caption",
|
||
"table caption", "title", "table", "text", "figure", "equation"]:
|
||
findLayout(lt)
|
||
|
||
# add box to figure layouts which has not text box
|
||
for i, lt in enumerate(
|
||
[lt for lt in lts if lt["type"] in ["figure", "equation"]]):
|
||
if lt.get("visited"):
|
||
continue
|
||
lt = deepcopy(lt)
|
||
del lt["type"]
|
||
lt["text"] = ""
|
||
lt["layout_type"] = "figure"
|
||
lt["layoutno"] = f"figure-{i}"
|
||
bxs.append(lt)
|
||
|
||
boxes.extend(bxs)
|
||
|
||
ocr_res = boxes
|
||
|
||
garbag_set = set()
|
||
for k in garbages.keys():
|
||
garbages[k] = Counter(garbages[k])
|
||
for g, c in garbages[k].items():
|
||
if c > 1:
|
||
garbag_set.add(g)
|
||
|
||
ocr_res = [b for b in ocr_res if b["text"].strip() not in garbag_set]
|
||
return ocr_res, page_layout
|
||
|
||
def forward(self, image_list, thr=0.7, batch_size=16):
|
||
return super().__call__(image_list, thr, batch_size)
|
||
|
||
class LayoutRecognizer4YOLOv10(LayoutRecognizer):
|
||
labels = [
|
||
"title",
|
||
"Text",
|
||
"Reference",
|
||
"Figure",
|
||
"Figure caption",
|
||
"Table",
|
||
"Table caption",
|
||
"Table caption",
|
||
"Equation",
|
||
"Figure caption",
|
||
]
|
||
|
||
def __init__(self, domain):
|
||
domain = "layout"
|
||
super().__init__(domain)
|
||
self.auto = False
|
||
self.scaleFill = False
|
||
self.scaleup = True
|
||
self.stride = 32
|
||
self.center = True
|
||
|
||
def preprocess(self, image_list):
|
||
inputs = []
|
||
new_shape = self.input_shape # height, width
|
||
for img in image_list:
|
||
shape = img.shape[:2]# current shape [height, width]
|
||
# Scale ratio (new / old)
|
||
r = min(new_shape[0] / shape[0], new_shape[1] / shape[1])
|
||
# Compute padding
|
||
new_unpad = int(round(shape[1] * r)), int(round(shape[0] * r))
|
||
dw, dh = new_shape[1] - new_unpad[0], new_shape[0] - new_unpad[1] # wh padding
|
||
dw /= 2 # divide padding into 2 sides
|
||
dh /= 2
|
||
ww, hh = new_unpad
|
||
img = np.array(cv2.cvtColor(img, cv2.COLOR_BGR2RGB)).astype(np.float32)
|
||
img = cv2.resize(img, new_unpad, interpolation=cv2.INTER_LINEAR)
|
||
top, bottom = int(round(dh - 0.1)) if self.center else 0, int(round(dh + 0.1))
|
||
left, right = int(round(dw - 0.1)) if self.center else 0, int(round(dw + 0.1))
|
||
img = cv2.copyMakeBorder(
|
||
img, top, bottom, left, right, cv2.BORDER_CONSTANT, value=(114, 114, 114)
|
||
) # add border
|
||
img /= 255.0
|
||
img = img.transpose(2, 0, 1)
|
||
img = img[np.newaxis, :, :, :].astype(np.float32)
|
||
inputs.append({self.input_names[0]: img, "scale_factor": [shape[1]/ww, shape[0]/hh, dw, dh]})
|
||
|
||
return inputs
|
||
|
||
def postprocess(self, boxes, inputs, thr):
|
||
thr = 0.08
|
||
boxes = np.squeeze(boxes)
|
||
scores = boxes[:, 4]
|
||
boxes = boxes[scores > thr, :]
|
||
scores = scores[scores > thr]
|
||
if len(boxes) == 0:
|
||
return []
|
||
class_ids = boxes[:, -1].astype(int)
|
||
boxes = boxes[:, :4]
|
||
boxes[:, 0] -= inputs["scale_factor"][2]
|
||
boxes[:, 2] -= inputs["scale_factor"][2]
|
||
boxes[:, 1] -= inputs["scale_factor"][3]
|
||
boxes[:, 3] -= inputs["scale_factor"][3]
|
||
input_shape = np.array([inputs["scale_factor"][0], inputs["scale_factor"][1], inputs["scale_factor"][0],
|
||
inputs["scale_factor"][1]])
|
||
boxes = np.multiply(boxes, input_shape, dtype=np.float32)
|
||
|
||
unique_class_ids = np.unique(class_ids)
|
||
indices = []
|
||
for class_id in unique_class_ids:
|
||
class_indices = np.where(class_ids == class_id)[0]
|
||
class_boxes = boxes[class_indices, :]
|
||
class_scores = scores[class_indices]
|
||
class_keep_boxes = nms(class_boxes, class_scores, 0.45)
|
||
indices.extend(class_indices[class_keep_boxes])
|
||
|
||
return [{
|
||
"type": self.label_list[class_ids[i]].lower(),
|
||
"bbox": [float(t) for t in boxes[i].tolist()],
|
||
"score": float(scores[i])
|
||
} for i in indices]
|
||
|