mirror of
https://gitlab.com/libeigen/eigen.git
synced 2025-07-16 01:51:51 +08:00
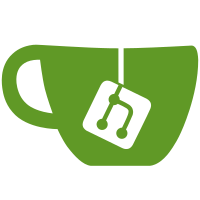
Instead we now have a custom thread pool that ensures that the functions are picked up by the threads in the pool in the order in which they are enqueued in the pool.
278 lines
9.3 KiB
C++
278 lines
9.3 KiB
C++
// This file is part of Eigen, a lightweight C++ template library
|
|
// for linear algebra.
|
|
//
|
|
// Copyright (C) 2014 Benoit Steiner <benoit.steiner.goog@gmail.com>
|
|
//
|
|
// This Source Code Form is subject to the terms of the Mozilla
|
|
// Public License v. 2.0. If a copy of the MPL was not distributed
|
|
// with this file, You can obtain one at http://mozilla.org/MPL/2.0/.
|
|
|
|
#define EIGEN_USE_THREADS
|
|
|
|
|
|
#include "main.h"
|
|
#include <iostream>
|
|
#include <Eigen/CXX11/Tensor>
|
|
|
|
using Eigen::Tensor;
|
|
|
|
|
|
static void test_multithread_elementwise()
|
|
{
|
|
Tensor<float, 3> in1(2,3,7);
|
|
Tensor<float, 3> in2(2,3,7);
|
|
Tensor<float, 3> out(2,3,7);
|
|
|
|
in1.setRandom();
|
|
in2.setRandom();
|
|
|
|
Eigen::ThreadPool tp(internal::random<int>(3, 11));
|
|
Eigen::ThreadPoolDevice thread_pool_device(&tp, internal::random<int>(3, 11));
|
|
out.device(thread_pool_device) = in1 + in2 * 3.14f;
|
|
|
|
for (int i = 0; i < 2; ++i) {
|
|
for (int j = 0; j < 3; ++j) {
|
|
for (int k = 0; k < 7; ++k) {
|
|
VERIFY_IS_APPROX(out(i,j,k), in1(i,j,k) + in2(i,j,k) * 3.14f);
|
|
}
|
|
}
|
|
}
|
|
}
|
|
|
|
|
|
static void test_multithread_compound_assignment()
|
|
{
|
|
Tensor<float, 3> in1(2,3,7);
|
|
Tensor<float, 3> in2(2,3,7);
|
|
Tensor<float, 3> out(2,3,7);
|
|
|
|
in1.setRandom();
|
|
in2.setRandom();
|
|
|
|
Eigen::ThreadPool tp(internal::random<int>(3, 11));
|
|
Eigen::ThreadPoolDevice thread_pool_device(&tp, internal::random<int>(3, 11));
|
|
out.device(thread_pool_device) = in1;
|
|
out.device(thread_pool_device) += in2 * 3.14f;
|
|
|
|
for (int i = 0; i < 2; ++i) {
|
|
for (int j = 0; j < 3; ++j) {
|
|
for (int k = 0; k < 7; ++k) {
|
|
VERIFY_IS_APPROX(out(i,j,k), in1(i,j,k) + in2(i,j,k) * 3.14f);
|
|
}
|
|
}
|
|
}
|
|
}
|
|
|
|
template<int DataLayout>
|
|
static void test_multithread_contraction()
|
|
{
|
|
Tensor<float, 4, DataLayout> t_left(30, 50, 37, 31);
|
|
Tensor<float, 5, DataLayout> t_right(37, 31, 70, 2, 10);
|
|
Tensor<float, 5, DataLayout> t_result(30, 50, 70, 2, 10);
|
|
|
|
t_left.setRandom();
|
|
t_right.setRandom();
|
|
|
|
// this contraction should be equivalent to a single matrix multiplication
|
|
typedef Tensor<float, 1>::DimensionPair DimPair;
|
|
Eigen::array<DimPair, 2> dims({{DimPair(2, 0), DimPair(3, 1)}});
|
|
|
|
typedef Map<Matrix<float, Dynamic, Dynamic, DataLayout>> MapXf;
|
|
MapXf m_left(t_left.data(), 1500, 1147);
|
|
MapXf m_right(t_right.data(), 1147, 1400);
|
|
Matrix<float, Dynamic, Dynamic, DataLayout> m_result(1500, 1400);
|
|
|
|
Eigen::ThreadPool tp(4);
|
|
Eigen::ThreadPoolDevice thread_pool_device(&tp, 4);
|
|
|
|
// compute results by separate methods
|
|
t_result.device(thread_pool_device) = t_left.contract(t_right, dims);
|
|
m_result = m_left * m_right;
|
|
|
|
for (ptrdiff_t i = 0; i < t_result.size(); i++) {
|
|
VERIFY(&t_result.data()[i] != &m_result.data()[i]);
|
|
if (fabs(t_result.data()[i] - m_result.data()[i]) >= 1e-4) {
|
|
std::cout << "mismatch detected: " << t_result.data()[i] << " vs " << m_result.data()[i] << std::endl;
|
|
assert(false);
|
|
}
|
|
}
|
|
}
|
|
|
|
template<int DataLayout>
|
|
static void test_contraction_corner_cases()
|
|
{
|
|
Tensor<float, 2, DataLayout> t_left(32, 500);
|
|
Tensor<float, 2, DataLayout> t_right(32, 28*28);
|
|
Tensor<float, 2, DataLayout> t_result(500, 28*28);
|
|
|
|
t_left = (t_left.constant(-0.5f) + t_left.random()) * 2.0f;
|
|
t_right = (t_right.constant(-0.6f) + t_right.random()) * 2.0f;
|
|
t_result = t_result.constant(NAN);
|
|
|
|
// this contraction should be equivalent to a single matrix multiplication
|
|
typedef Tensor<float, 1>::DimensionPair DimPair;
|
|
Eigen::array<DimPair, 1> dims{{DimPair(0, 0)}};
|
|
|
|
typedef Map<Matrix<float, Dynamic, Dynamic, DataLayout>> MapXf;
|
|
MapXf m_left(t_left.data(), 32, 500);
|
|
MapXf m_right(t_right.data(), 32, 28*28);
|
|
Matrix<float, Dynamic, Dynamic, DataLayout> m_result(500, 28*28);
|
|
|
|
Eigen::ThreadPool tp(12);
|
|
Eigen::ThreadPoolDevice thread_pool_device(&tp, 12);
|
|
|
|
// compute results by separate methods
|
|
t_result.device(thread_pool_device) = t_left.contract(t_right, dims);
|
|
m_result = m_left.transpose() * m_right;
|
|
|
|
for (ptrdiff_t i = 0; i < t_result.size(); i++) {
|
|
assert(!std::isnan(t_result.data()[i]));
|
|
if (fabs(t_result.data()[i] - m_result.data()[i]) >= 1e-4) {
|
|
std::cout << "mismatch detected at index " << i << " : " << t_result.data()[i] << " vs " << m_result.data()[i] << std::endl;
|
|
assert(false);
|
|
}
|
|
}
|
|
|
|
t_left.resize(32, 1);
|
|
t_left = (t_left.constant(-0.5f) + t_left.random()) * 2.0f;
|
|
t_result.resize (1, 28*28);
|
|
t_result = t_result.constant(NAN);
|
|
t_result.device(thread_pool_device) = t_left.contract(t_right, dims);
|
|
new(&m_left) MapXf(t_left.data(), 32, 1);
|
|
m_result = m_left.transpose() * m_right;
|
|
for (ptrdiff_t i = 0; i < t_result.size(); i++) {
|
|
assert(!std::isnan(t_result.data()[i]));
|
|
if (fabs(t_result.data()[i] - m_result.data()[i]) >= 1e-4) {
|
|
std::cout << "mismatch detected: " << t_result.data()[i] << " vs " << m_result.data()[i] << std::endl;
|
|
assert(false);
|
|
}
|
|
}
|
|
|
|
t_left.resize(32, 500);
|
|
t_right.resize(32, 4);
|
|
t_left = (t_left.constant(-0.5f) + t_left.random()) * 2.0f;
|
|
t_right = (t_right.constant(-0.6f) + t_right.random()) * 2.0f;
|
|
t_result.resize (500, 4);
|
|
t_result = t_result.constant(NAN);
|
|
t_result.device(thread_pool_device) = t_left.contract(t_right, dims);
|
|
new(&m_left) MapXf(t_left.data(), 32, 500);
|
|
new(&m_right) MapXf(t_right.data(), 32, 4);
|
|
m_result = m_left.transpose() * m_right;
|
|
for (ptrdiff_t i = 0; i < t_result.size(); i++) {
|
|
assert(!std::isnan(t_result.data()[i]));
|
|
if (fabs(t_result.data()[i] - m_result.data()[i]) >= 1e-4) {
|
|
std::cout << "mismatch detected: " << t_result.data()[i] << " vs " << m_result.data()[i] << std::endl;
|
|
assert(false);
|
|
}
|
|
}
|
|
|
|
t_left.resize(32, 1);
|
|
t_right.resize(32, 4);
|
|
t_left = (t_left.constant(-0.5f) + t_left.random()) * 2.0f;
|
|
t_right = (t_right.constant(-0.6f) + t_right.random()) * 2.0f;
|
|
t_result.resize (1, 4);
|
|
t_result = t_result.constant(NAN);
|
|
t_result.device(thread_pool_device) = t_left.contract(t_right, dims);
|
|
new(&m_left) MapXf(t_left.data(), 32, 1);
|
|
new(&m_right) MapXf(t_right.data(), 32, 4);
|
|
m_result = m_left.transpose() * m_right;
|
|
for (ptrdiff_t i = 0; i < t_result.size(); i++) {
|
|
assert(!std::isnan(t_result.data()[i]));
|
|
if (fabs(t_result.data()[i] - m_result.data()[i]) >= 1e-4) {
|
|
std::cout << "mismatch detected: " << t_result.data()[i] << " vs " << m_result.data()[i] << std::endl;
|
|
assert(false);
|
|
}
|
|
}
|
|
}
|
|
|
|
template<int DataLayout>
|
|
static void test_multithread_contraction_agrees_with_singlethread() {
|
|
int contract_size = internal::random<int>(1, 5000);
|
|
|
|
Tensor<float, 3, DataLayout> left(internal::random<int>(1, 80),
|
|
contract_size,
|
|
internal::random<int>(1, 100));
|
|
|
|
Tensor<float, 4, DataLayout> right(internal::random<int>(1, 25),
|
|
internal::random<int>(1, 37),
|
|
contract_size,
|
|
internal::random<int>(1, 51));
|
|
|
|
left.setRandom();
|
|
right.setRandom();
|
|
|
|
// add constants to shift values away from 0 for more precision
|
|
left += left.constant(1.5f);
|
|
right += right.constant(1.5f);
|
|
|
|
typedef Tensor<float, 1>::DimensionPair DimPair;
|
|
Eigen::array<DimPair, 1> dims({{DimPair(1, 2)}});
|
|
|
|
Eigen::ThreadPool tp(internal::random<int>(2, 11));
|
|
Eigen::ThreadPoolDevice thread_pool_device(&tp, internal::random<int>(2, 11));
|
|
|
|
Tensor<float, 5, DataLayout> st_result;
|
|
st_result = left.contract(right, dims);
|
|
|
|
Tensor<float, 5, DataLayout> tp_result(st_result.dimensions());
|
|
tp_result.device(thread_pool_device) = left.contract(right, dims);
|
|
|
|
VERIFY(dimensions_match(st_result.dimensions(), tp_result.dimensions()));
|
|
for (ptrdiff_t i = 0; i < st_result.size(); i++) {
|
|
// if both of the values are very small, then do nothing (because the test will fail
|
|
// due to numerical precision issues when values are small)
|
|
if (fabs(st_result.data()[i] - tp_result.data()[i]) >= 1e-4) {
|
|
VERIFY_IS_APPROX(st_result.data()[i], tp_result.data()[i]);
|
|
}
|
|
}
|
|
}
|
|
|
|
|
|
static void test_memcpy() {
|
|
|
|
for (int i = 0; i < 5; ++i) {
|
|
const int num_threads = internal::random<int>(3, 11);
|
|
Eigen::ThreadPool tp(num_threads);
|
|
Eigen::ThreadPoolDevice thread_pool_device(&tp, num_threads);
|
|
|
|
const int size = internal::random<int>(13, 7632);
|
|
Tensor<float, 1> t1(size);
|
|
t1.setRandom();
|
|
std::vector<float> result(size);
|
|
thread_pool_device.memcpy(&result[0], t1.data(), size*sizeof(float));
|
|
for (int i = 0; i < size; i++) {
|
|
VERIFY_IS_EQUAL(t1(i), result[i]);
|
|
}
|
|
}
|
|
}
|
|
|
|
|
|
static void test_multithread_random()
|
|
{
|
|
Eigen::ThreadPool tp(2);
|
|
Eigen::ThreadPoolDevice device(&tp, 2);
|
|
Tensor<float, 1> t(1 << 20);
|
|
t.device(device) = t.random<Eigen::internal::NormalRandomGenerator<float>>();
|
|
}
|
|
|
|
|
|
void test_cxx11_tensor_thread_pool()
|
|
{
|
|
CALL_SUBTEST(test_multithread_elementwise());
|
|
CALL_SUBTEST(test_multithread_compound_assignment());
|
|
|
|
CALL_SUBTEST(test_multithread_contraction<ColMajor>());
|
|
CALL_SUBTEST(test_multithread_contraction<RowMajor>());
|
|
|
|
CALL_SUBTEST(test_multithread_contraction_agrees_with_singlethread<ColMajor>());
|
|
CALL_SUBTEST(test_multithread_contraction_agrees_with_singlethread<RowMajor>());
|
|
|
|
// Exercise various cases that have been problematic in the past.
|
|
CALL_SUBTEST(test_contraction_corner_cases<ColMajor>());
|
|
CALL_SUBTEST(test_contraction_corner_cases<RowMajor>());
|
|
|
|
CALL_SUBTEST(test_memcpy());
|
|
|
|
CALL_SUBTEST(test_multithread_random());
|
|
}
|